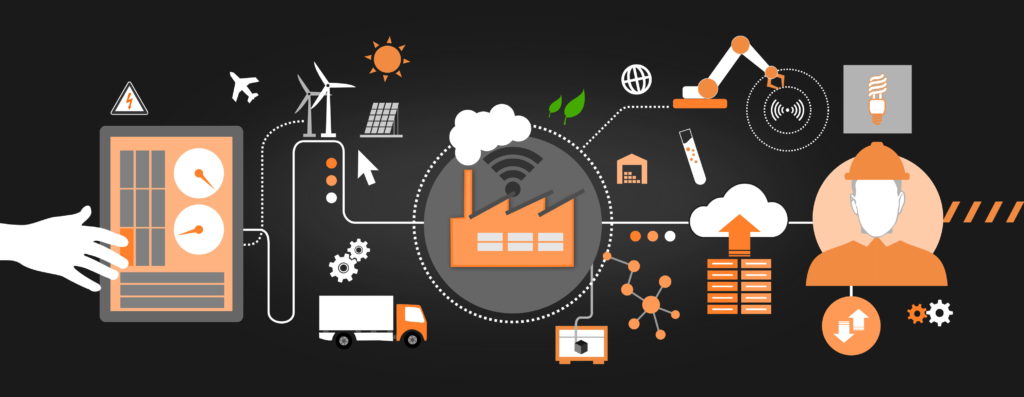
IoT Analytics: Telling It Inside Out / Building The Complete Picture
At an estimated USD 3.9 Tn Industry 4.0 is being seen as the domain with the most to gain from the Internet of Things (IoT). Manufacturing firms world over are using IoT to improve operational efficiencies, to automate and to innovate to discover additional sources of revenue with new business models. Enterprises are realizing that data analytics and connected devices will be required to enjoy higher efficiencies and process improvements.
With the advent of IoT, Industry 4.0 has taken this digitally driven transformation to another level via interconnectivity and access to real-time data. A variety of sensors, dramatic increases in storage capacity and processing power, real-time analytics of unprecedented sophistication, and the ability to translate that data into meaningful action – are all helping organizations predictively maintain equipment and operations in order to optimize performance. The data analytics being derived from IoT sensors is helping companies forecast potential issues, minimize downtime and also eliminate any guesswork from preventive maintenance. IoT analytics is being used today to structure, process and analyze data and churn out invaluable insights and support better decisions.
One key advantage that the trifecta of IoT, big data and advanced analytics brings is that it enables systemic interoperability and collaboration between diverse teams and operations delivering cost and efficiency benefits. IoT analytics are playing a crucial role in modern industrial systems, adding an information layer to the conventional methods for data collection, storage and analysis.
IoT analytics for preventive maintenance
Until recently, factory managers and machine operators carried out scheduled maintenance manually and regularly repaired machine parts to prevent downtime. This process was time consuming and counter-productive, and despite the time invested most of the predictive maintenance steps taken were ineffective. Implementing IoT to monitor asset health, optimize maintenance schedules, and gaining real-time alerts to operational risks, allowed enterprises to lower service costs, maximize uptime, and improve production throughput.
They are now building blueprints of a connected system that includes equipment and sensors, business systems, communication protocols, gateways, cloud, predictive analytics, and visualization. This allows IoT sensor data to be captured and used for predictive analytics that can be applied to the machine data and predict conditions of upcoming failure. A dashboard for predictive analytics processes operational data allows engineers to address actionable insights and take corrective action. Rule-based predictive maintenance allows enterprises to bypass the need for large historical data sets – at least initially – or advanced machine learning algorithms, instead giving them faster results and a step into advanced analytics.
Advanced analytics with predictive alerts and automated root cause analysis can be applied at a later phase, and that’s when historical data can be used to accurately predict issues. IoT analytics help organizations enhance overall efficiency, improve safety procedures and apply quick fixes to maintenance problems. Machine learning and AI technologies are giving more impetus by helping enterprises connect disparate data sources and gain insights for forecasting future performance.
Making grids smarter with IoT analytics
The transformation of electrical grids into smart grids is perhaps one of the major technological challenges, and achievements, of the past decade and also one of the key growth areas for IoT analytics. Smart-home technologies and the corresponding analytics are an integral part of many use cases in this field. Smart grid solutions based on IoT technology are playing a huge role in energy conservation by connecting disparate platforms in home automation, building and infrastructure automation, as well as in transmission and distribution systems.
Smart grids collect much more data than the manual energy meter reading system, which warrants the need for data analysis and highly realistic consumption forecasts that take a multitude of variables into account. Smart grid analytics are expanding because there are exponentially more data available thanks to IoT sensors to develop analytical models that could even predict future failures. What makes the IoT smart grid better is two-way communication between connected devices and hardware that can sense and respond to user demands, and also gather performance data and feed it back to the supplier offering deep analytics and insights. Data analytics combined with grid visualization can lead to better situational awareness, preventive maintenance and fault detection, as well advanced metering infrastructure and the security of the power system.
IoT sensors connect to the gateway, which in turn connects to the cloud and enables access to sensor data remotely via mobile devices. Sensors also collect energy consumption data on real-time from devices, and this data is analyzed by the gateway, which then escalates the necessary output or command message (like a utility command, an alert, HVAC control etc) to the control system. IoT devices also help analyze energy utilization of each device, which aids the user in managing device up/down time. Enterprises consuming energy can access historical data from the cloud, derive insights and accordingly optimize their consumption of energy.
The IoT has allowed companies to move towards a new way of doing business by applying it to various processes in order to enhance productivity and efficiency. But the real value of IoT lies in using the data from the cloud and the edge to get better analytics and derive insights from raw data. Analytics can play a much broader role and influence business practices, predictions, ROI, decision-making and more. Combining the IoT with advanced analytics takes businesses to the next level by offering transparency into business operations, insight into market trends and highlighting opportunities for improving the business.
We, at Tibil, understand the immense potential data holds for business and help enterprises harness its power fully, helping them achieve larger business goals through reliable and intelligent management of data. Our IoT Data Analytics services help you leverage your IoT device data to create immersive and insightful reports that can be combined with contextual data. We drive big data analytics, predictive analytics and customer analytics using new generation data analytics technologies, ML/AI and industry-grade statistical models to deliver advanced, real-time analytics.
Related Posts
AI Toolbox: Creative Content Beyond ChatGPT & BARD
Introduction: In the dynamic landscape of artificial intelligence (AI), ChatGPT and BARD have garnered significant attention for their capabilities in natural language processing and music composition. However, a rich tapestry of AI tools exists...
Ethical Considerations in Generative AI: Ensuring Fair and Responsible Data Analytics
Introduction Generative AI, a transformative subset of artificial intelligence, is revolutionizing data analytics and machine learning. It empowers machines to generate data, images, and text that mimic human creativity. While the potential of...
Building a Better Future with Digital Public Goods
The world is on the cusp of digitization! In this era of digitization, we have transformed the way we communicate, interact, and access information. It has not only changed our personal lives but also has brought an evident transformation in the...
The Role of Artificial Intelligence in Cyber Security
In an era characterised by rapid technological advancements and increasing digitalisation, the field of cyber security faces an escalating and ever-evolving threat landscape. As cyber threats become more sophisticated, organisations must employ...
TinyML: The Future of Edge AI
Artificial intelligence (AI) has been a hot topic in recent years and with good reason. AI has the potential to transform countless industries and improve our lives in numerous ways. However, as powerful as AI can be, it also requires a lot of...
The Evolution of Federated Learning
Uber settled an inquiry into a data breach that exposed the personal data of more than 5,00,000 drivers in 2016 by paying $148 million. A GDPR breach resulted in a $57 million fine for Google in 2020. On-growing data privacy and data breach issues...
Subscribe To Our Newsletter
Lorem ipsum dolor sit amet, consectetuer adipiscing elit. Aenean commodo ligula eget dolor. Aenean massa. Cum sociis natoque penatibus et magnis dis parturient montes, nascetur ridiculus mus

Company
About us
Careers
Contact
Awards
Blog
Offerings
Strategy & Consulting
Managed Services
Solutions
Digital Public Goods
Solutions
Data Solutions
Industry Solutions