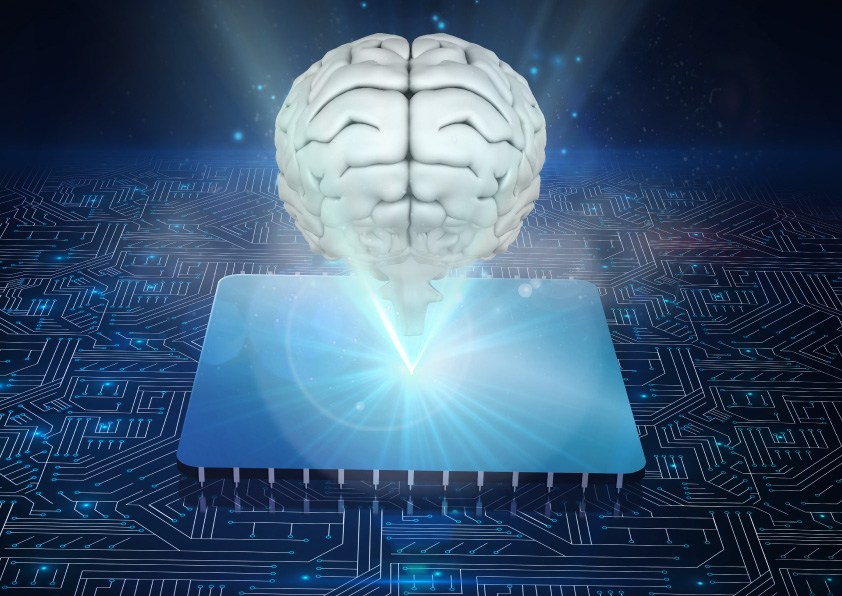
TinyML: The Future of Edge AI
Artificial intelligence (AI) has been a hot topic in recent years and with good reason. AI has the potential to transform countless industries and improve our lives in numerous ways. However, as powerful as AI can be, it also requires a lot of computing power, which can be a major limiting factor. This is where TinyML comes in.
TinyML is a branch of machine learning that focuses on making AI models small and efficient enough to run on low-power devices, such as microcontrollers and single-board computers. The goal of TinyML is to bring the power of AI to the edge, allowing devices to make decisions and respond to their environment without the need for a connection to a central server.
One of the main benefits of TinyML is that it can enable devices to operate more independently and make decisions in real time. This can lead to a wide range of applications, from smart homes and wearable devices to industrial automation and autonomous vehicles. For example, a smart home thermostat could use TinyML to automatically adjust the temperature based on the presence of people in the room, without sending data to the cloud for processing.
Another key advantage of TinyML is that it can greatly reduce the amount of data that needs to be sent to the cloud, reducing bandwidth and privacy concerns. By processing data locally, TinyML can also help to ensure that devices continue to function even when they are offline.
While TinyML is still a relatively new field, it has already shown tremendous potential and is poised to become a major player in the AI space. With the growing demand for low-power, connected devices, TinyML is set to play a crucial role in shaping the future of the Internet of Things (IoT) and beyond.
How does it work?
TinyML functions by operating machine learning models on low-power devices, like microcontrollers and single-board computers, rather than relying on robust servers and cloud infrastructure. The key to enabling this is by reducing the size and complexity of the models, allowing them to operate efficiently on limited hardware.
To accomplish this, TinyML usually employs methods such as quantization, pruning, and compression to decrease the models’ size. Quantization entails lowering the precision of the model’s parameters, making it possible to store and process data more efficiently. Pruning involves eliminating unimportant or redundant sections of the model, reducing its size and complexity without sacrificing accuracy. Compression utilizes algorithms to further minimize the model’s size and improve its efficiency.
Once the model has been optimized for size and efficiency, it can be deployed on the edge device and employed for various tasks, such as object detection, speech recognition, and gesture recognition. The device processes the data locally, utilizing the TinyML model to make decisions and respond to the environment in real time. This eliminates the necessity for a constant connection to the cloud and reduces latency, making it feasible to utilize AI in applications where low latency and responsiveness are crucial.
Benefits of TinyML
Reduced latency: With TinyML, machine learning algorithms can run on-device, reducing the need for sending data to remote servers for processing, which can lead to lower latency and faster response time.
Enhanced privacy and security: As machine learning algorithms run locally on devices, they don’t need to send data to remote servers, which can enhance data privacy and security.
Energy efficiency: TinyML models are designed to run on small, low-power devices, which means they consume less energy and can run for longer periods without requiring a recharge.
Improved reliability: TinyML models can continue to function even when there’s no network connectivity, which can be critical in environments where a consistent network connection is not guaranteed.
Real-time processing: TinyML enables real-time processing of data on devices, which can be important in use cases where fast decision-making is critical, such as in autonomous vehicles or medical devices.
Cost-effective: TinyML models are lightweight and designed to run on low-cost devices, making them an affordable solution for businesses and individuals looking to deploy machine learning applications on a large scale.
TinyML has a wide range of potential use cases, some of which include:
TinyML can be applied to various industries including wearables, smart homes, industrial automation, healthcare, agriculture, and environmental monitoring. It allows wearable devices to perform tasks such as gesture recognition, heart rate monitoring, and sleep tracking. In smart homes, TinyML enables devices to respond to their environment without sending data to the cloud.
In industrial automation, TinyML can be used for object detection, machine vision, and predictive maintenance. Healthcare devices can leverage TinyML for continuous monitoring of vital signs and early detection of potential health issues.
TinyML can also be used in agriculture to optimize irrigation and fertilization and in environmental monitoring systems for real-time decision-making without a constant connection to the cloud. As a result of its ability to run AI models on low-power devices, TinyML has the potential to significantly impact the IoT and beyond.
Here are some of the companies that are actively involved in the development and deployment of TinyML:
Several technology companies are investing in TinyML for deploying AI models on low-power devices. Google is supporting TinyML through its Edge TPU platform, while Apple is already utilizing TinyML in its devices such as Apple Watch and AirPods for tasks like gesture recognition and voice command processing.
Amazon is using TinyML in its devices such as Echo Dot and Fire TV Stick for speech recognition and voice command processing.
Qualcomm, a leading provider of mobile processors and modems, is investing in TinyML to enable AI on various devices, including smartphones and IoT devices.
ARM and MediaTek, both leading providers of microprocessors, are developing TinyML-optimized chips for running AI models on low-power devices. Finally, Xnor.ai is a startup that is dedicated to developing TinyML models and tools for deploying them on low-power devices.
In conclusion,
TinyML is a game-changer in the world of AI, providing a way to bring the power of machine learning to the edge. With its ability to process data locally, respond in real time, and reduce bandwidth and privacy concerns, TinyML is set to play a crucial role in shaping the future of the IoT and beyond. So, keep an eye out for TinyML – it’s the future of AI, and it’s here to stay.
Related Posts
AI Toolbox: Creative Content Beyond ChatGPT & BARD
Introduction: In the dynamic landscape of artificial intelligence (AI), ChatGPT and BARD have garnered significant attention for their capabilities in natural language processing and music composition. However, a rich tapestry of AI tools exists...
Ethical Considerations in Generative AI: Ensuring Fair and Responsible Data Analytics
Introduction Generative AI, a transformative subset of artificial intelligence, is revolutionizing data analytics and machine learning. It empowers machines to generate data, images, and text that mimic human creativity. While the potential of...
Building a Better Future with Digital Public Goods
The world is on the cusp of digitization! In this era of digitization, we have transformed the way we communicate, interact, and access information. It has not only changed our personal lives but also has brought an evident transformation in the...
The Role of Artificial Intelligence in Cyber Security
In an era characterised by rapid technological advancements and increasing digitalisation, the field of cyber security faces an escalating and ever-evolving threat landscape. As cyber threats become more sophisticated, organisations must employ...
The Evolution of Federated Learning
Uber settled an inquiry into a data breach that exposed the personal data of more than 5,00,000 drivers in 2016 by paying $148 million. A GDPR breach resulted in a $57 million fine for Google in 2020. On-growing data privacy and data breach issues...
Chatbots and What They Can Do in the Manufacturing Industry
In recent years the development of chatbots and conversational AI has progressed extremely rapidly. What was originally used primarily for B2C customer interactions is today used in an interesting variety of applications. In the manufacturing...
Subscribe To Our Newsletter
Lorem ipsum dolor sit amet, consectetuer adipiscing elit. Aenean commodo ligula eget dolor. Aenean massa. Cum sociis natoque penatibus et magnis dis parturient montes, nascetur ridiculus mus

Company
About us
Careers
Contact
Awards
Blog
Offerings
Strategy & Consulting
Managed Services
Solutions
Digital Public Goods
Solutions
Data Solutions
Industry Solutions