Big Data Analytics for Education Sector
Superior business intelligence & analytics
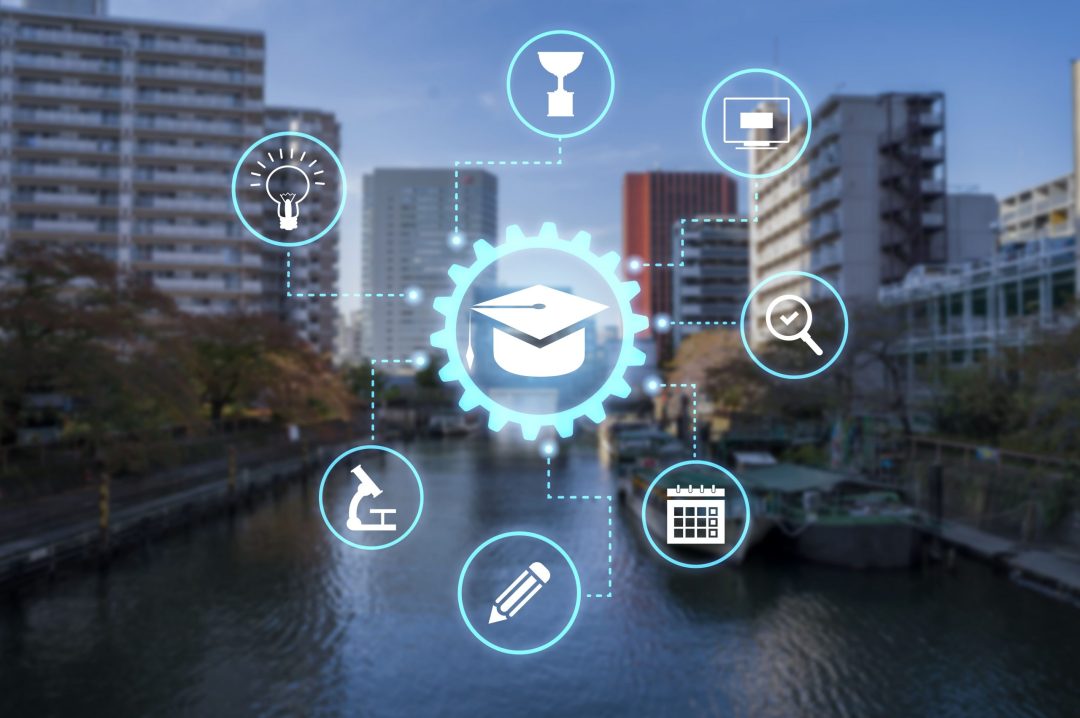
Big Data Analytics Defines the Future of Education Industry
“Higher studies” or professional education is an area that needs constant evaluation and transformation to ensure that the studies that come out of it are fully equipped to meet the challenges of a highly dynamic workplace. Technology is a key driver in ensuring that the education system remains state of the art. Examples of different types of technologies used in education are mobile devices, teleconferences, and remote access systems, educational platforms and services. All of these ensure that students, teachers, academic faculty, evaluation specialists, researchers and decision-makers in education interact with each other, in an effort to impact and improve teaching and learning but also to realistically reflect in the learning stage the usage of modern technologies used in real settings. The interaction with these technologies generates large amounts of data that range from an individual access log file to an institutional-level activity.
Despite all efforts in this direction, the educational systems are not yet fully prepared to cope with and exploit technology for continuous quality improvement purposes. The need to govern these challenges within the education industry is currently extremely important, and therefore, attention has been paid to different approaches such as big data and analytics.
Tibil helped an innovative, technology-driven non-profit organization embark on creating open access to learning opportunities for millions by building advanced analytics capabilities on the apps and portals they were developing.
Adaptive Learning
A highly configurable and user-friendly smart e-learning platform aimed at empowering the teachers in schools to improve the academic proficiency of their students. By using an Adaptive Learning platform in a modern education system, the students will be protagonists and leaders of their own training. The learners will enter the e-learning platform and they will have the opportunity to choose their learning path according to the results obtained in the evaluations. Therefore, the platform can offer each student different content that adapts to their learning needs, helps them identify and work on their shortcomings or serves to address questions or topics that are not clear at all.
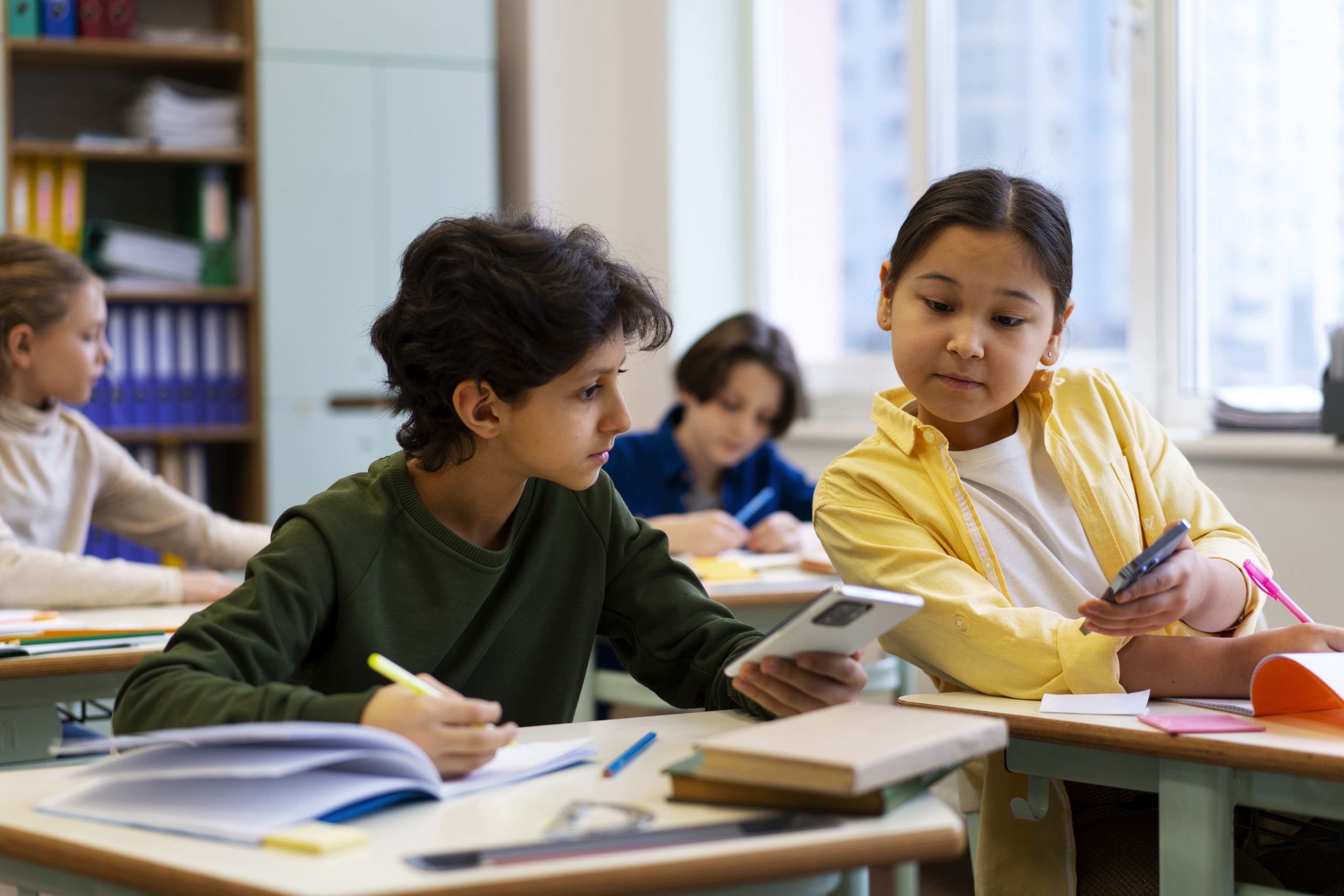
The Adaptive learning platform uses ML to not only make connections, but predictions when it comes to the progress and risks of learners, but also make it a versatile tool for any educational content provider. Unlike other platforms that may only focus on teaching a subject or model, the software can be tailored to improve math skills. This allows for a much faster training and learning curve that bases progress on skill mastery.
The platform is built on the idea that not every student will understand every concept at the same time. To address this fact, we use real-time technology. This technology assists students with exercises in practice, assessment, and comprehension. This approach is advantageous for colleges and universities because it brings together performance-driven curriculums with traditional critical thinking concepts using online tools. The result is a software that does everything from assessing skills to predicting student performance while closing any learning gaps.
Academic Analytics
The focus of academic analytics includes reporting, modelling, analysis and decision support functions across university and campus services. Examples of these kinds of services include, but are not limited to admission, advising, financing, academic counseling, enrolment, and administration. Academic analytics can be compared to business intelligence and includes decision-making support relating to university and campus services.
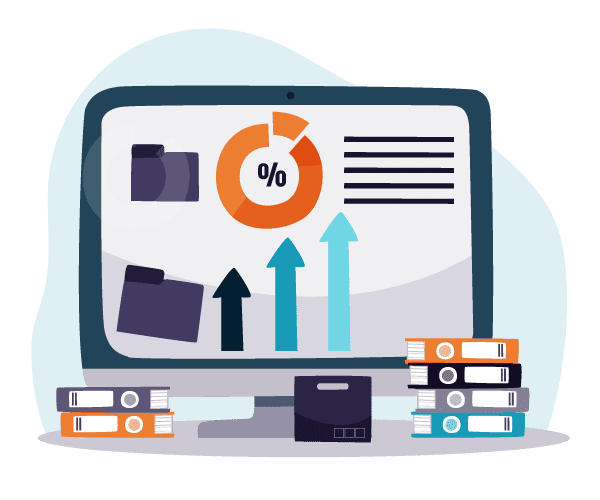
In most of the cases, Academic Analytics have been used to provide actionable insights and support single or isolated decisions. In fact, Academic Analytics is a main part of the quality improvement process and can be beneficial in multiple ways in the steps of the improvement cycle. In the early steps of the cycle, it can support decision-makers to identify what is possible or necessary to improve. In the following steps, academic analytics can support decisions about choosing appropriate actions through predictions and by providing “what if” scenarios using the need-driven approach. Academic analytics (through dashboards and reports) can be used to monitor the ongoing processes and support decisions concerning eventual adjustments. At the end of the quality improvement cycle, academic analytics can support performing evaluations of the intervention’s impact demonstrating the hidden connections between actions and events.
© Tibil Solutions 2024. All rights reserved